Pandas cheat sheet¶¶
- Pandas Cheat Sheet Github
- Pandas Cheat Sheet For Data Analysis
- Pandas Cheat Sheet Data Science
- Python For Data Science Cheat Sheet
NumPy / SciPy / Pandas Cheat Sheet Select column. Select row by label. Return DataFrame index. Delete given row or column. Pass axis=1 for columns. Reindex df1 with index of df2. Reset index, putting old index in column named index. Change DataFrame index, new indecies set to NaN. Show first n rows. Show last n rows. Python For Data Science Cheat Sheet Pandas Basics Learn Python for Data Science Interactively at www.DataCamp.com Pandas DataCamp Learn Python for Data Science Interactively Series DataFrame 4 Index 7-5 3 d c b A one-dimensional labeled array a capable of holding any data type Index Columns A two-dimensional labeled data structure with columns. Pandas cheat sheet will help you quickly look for methods you have already learned, and it can come in handy even if you are going for an exam or interview. We have collected and grouped all the commands used frequently in the Pandas by a data analyst for easy detection. In this Pandas cheat sheet, we will use the following shorthand for. To excel data analysis/data science/machine learningin Python, Pandasis a library you need to master. Here is a cheat sheetof some of the most used syntax that you probably don’t want to miss. The Pandas package is the most imperativetool in Data Science and Analysis working in Python nowadays. Pandas Cheat Sheet: top 35 commands and operations Pandas is one of the most popular tools for data analysis in Python. This open-source library is the backbone of many data projects and is used for data cleaning and data manipulation. With Pandas, you gain greater control over complex data sets.
Pandas is Python Data Analysis library. Series and Dataframes are major data structures in Pandas. Pandas is built on top of NumPy arrays.
ToC
- Series
- DataFrames
- Slicing and dicing DataFrames
- Conditional selection
- Operations on DataFrames
- DataFrame index
Pandas Cheat Sheet Github
Series¶¶
Series is 1 dimensional data structure. It is similar to numpy array, but each data point has a label in the place of an index.
Create a series¶¶
Thus Series can have different datatypes.
Operations on series¶¶
You can add, multiply and other numerical opertions on Series just like on numpy arrays.
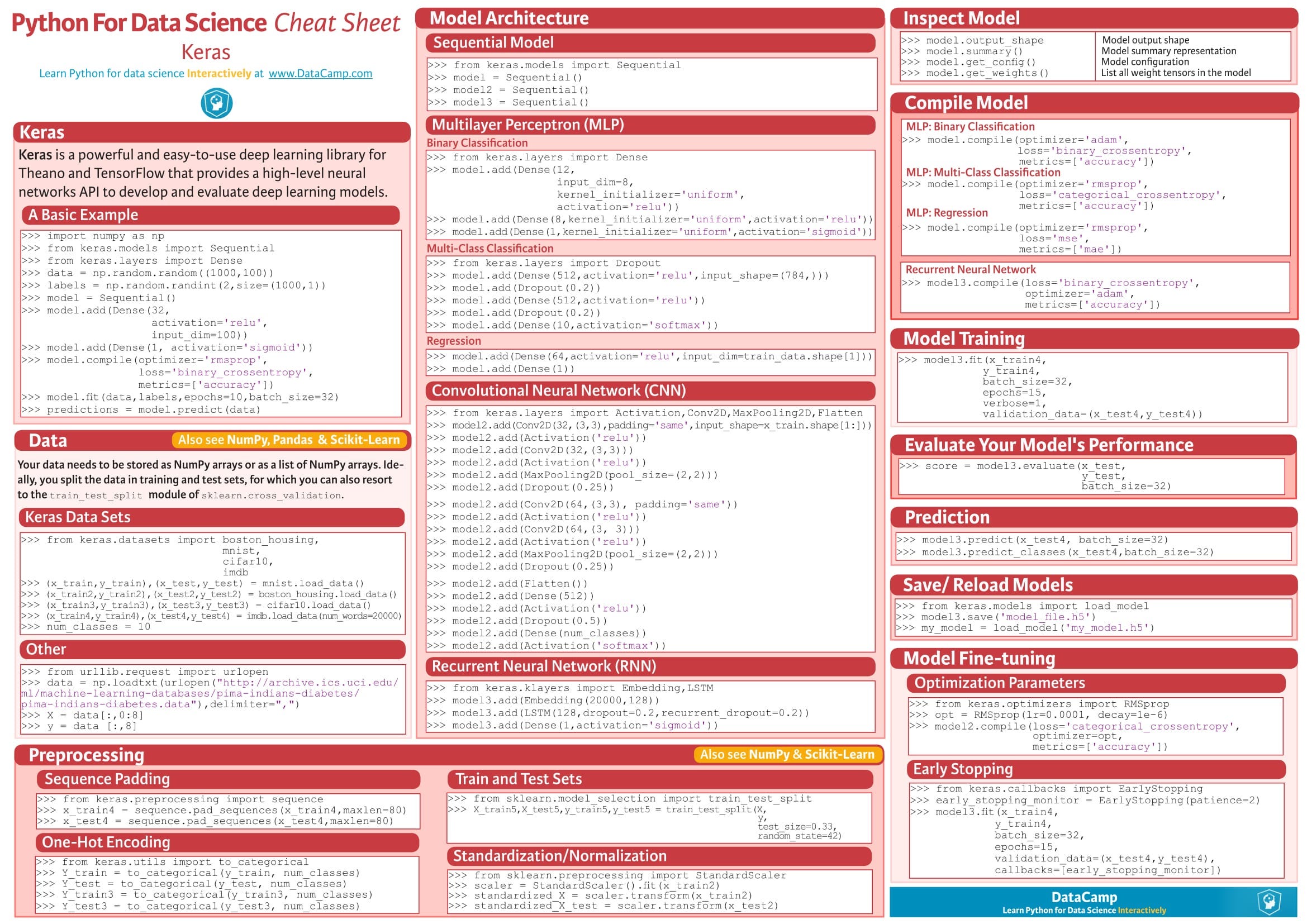
When labels dont match, it puts a nan. Thus when two series are added, you may or may not get the same number of elements
DataFrames¶¶
Creating dataFrames¶¶
Pandas DataFrames are built on top of Series. It looks similar to a NumPy array, but has labels for both columns and rows.
reliability | cost | competition | halflife | |
---|---|---|---|---|
Car1 | 0.134302 | 0.625207 | 0.970981 | 0.717605 |
Car2 | 0.713766 | 0.773182 | 0.059689 | 0.450899 |
Car3 | 0.058990 | 0.904301 | 0.431487 | 0.087683 |
Car4 | 0.509891 | 0.501037 | 0.244279 | 0.763135 |
Slicing and dicing DataFrames¶¶
You can access DataFrames similar to Series and slice it similar to NumPy arrays
Access columns¶¶
Accessing using index number¶¶
If you don’t know the labels, but know the index like in an array, use iloc
and pass the index number.
Dicing DataFrames¶¶
Dicing using labels > use DataFrameObj.loc[[row_labels],[col_labels]]
cost | competition | |
---|---|---|
Car2 | 0.935368 | 0.719570 |
Car3 | 0.659950 | 0.605077 |
cost | competition | |
---|---|---|
Car2 | 0.935368 | 0.719570 |
Car3 | 0.659950 | 0.605077 |
Conditional selection¶¶
When running a condition on a DataFrame, you are returned a Bool dataframe.
reliability | cost | competition | halflife | |
---|---|---|---|---|
Car1 | 0.776415 | 0.435083 | 0.236151 | 0.169087 |
Car2 | 0.790403 | 0.987459 | 0.370570 | 0.734146 |
Car3 | 0.884783 | 0.233803 | 0.691639 | 0.725398 |
Car4 | 0.693038 | 0.716824 | 0.766937 | 0.490821 |
reliability | cost | competition | halflife | |
---|---|---|---|---|
Car3 | 0.884783 | 0.233803 | 0.691639 | 0.725398 |
Chaining conditions¶¶
In a Pythonic way, you can chain conditions
Multiple conditions¶¶
You can select dataframe elements with multiple conditions. Note cannot use Python and
, or
. Instead use &
, |
reliability | cost | competition | halflife | |
---|---|---|---|---|
Car1 | 0.776415 | 0.435083 | 0.236151 | 0.169087 |
Car2 | 0.790403 | 0.987459 | 0.370570 | 0.734146 |
reliability | cost | competition | halflife | |
---|---|---|---|---|
Car1 | 0.776415 | 0.435083 | 0.236151 | 0.169087 |
Car2 | 0.790403 | 0.987459 | 0.370570 | 0.734146 |
Car3 | 0.884783 | 0.233803 | 0.691639 | 0.725398 |
Operations on DataFrames¶¶
Adding new columns¶¶
Create new columns just like adding a kvp to a dictionary.
reliability | cost | competition | halflife | full_life | |
---|---|---|---|---|---|
Car1 | 0.134302 | 0.625207 | 0.970981 | 0.717605 | 1.435210 |
Car2 | 0.713766 | 0.773182 | 0.059689 | 0.450899 | 0.901799 |
Car3 | 0.058990 | 0.904301 | 0.431487 | 0.087683 | 0.175366 |
Car4 | 0.509891 | 0.501037 | 0.244279 | 0.763135 | 1.526270 |
Dropping rows and columns¶¶
Row labels are axis = 0
and columns are axis = 1
reliability | cost | competition | halflife | |
---|---|---|---|---|
Car1 | 0.134302 | 0.625207 | 0.970981 | 0.717605 |
Car2 | 0.713766 | 0.773182 | 0.059689 | 0.450899 |
Car3 | 0.058990 | 0.904301 | 0.431487 | 0.087683 |
Car4 | 0.509891 | 0.501037 | 0.244279 | 0.763135 |
reliability | cost | competition | halflife | full_life | |
---|---|---|---|---|---|
Car1 | 0.134302 | 0.625207 | 0.970981 | 0.717605 | 1.435210 |
Car2 | 0.713766 | 0.773182 | 0.059689 | 0.450899 | 0.901799 |
Car4 | 0.509891 | 0.501037 | 0.244279 | 0.763135 | 1.526270 |
reliability | cost | competition | halflife | full_life | |
---|---|---|---|---|---|
Car1 | 0.134302 | 0.625207 | 0.970981 | 0.717605 | 1.43521 |
Car4 | 0.509891 | 0.501037 | 0.244279 | 0.763135 | 1.52627 |
DataFrame Index¶¶
So far, Car1
, Car2
.. is the index for rows. If you would like to set a different column as an index, use set_index
. If you want to make index as a column rather, and use numerals for index, use reset_index
Set index¶¶
reliability | cost | competition | halflife | car_names | |
---|---|---|---|---|---|
Car1 | 0.776415 | 0.435083 | 0.236151 | 0.169087 | altima |
Car2 | 0.790403 | 0.987459 | 0.370570 | 0.734146 | outback |
Car3 | 0.884783 | 0.233803 | 0.691639 | 0.725398 | taurus |
Car4 | 0.693038 | 0.716824 | 0.766937 | 0.490821 | mustang |
reliability | cost | competition | halflife | car_names | |
---|---|---|---|---|---|
car_names | |||||
altima | 0.776415 | 0.435083 | 0.236151 | 0.169087 | altima |
outback | 0.790403 | 0.987459 | 0.370570 | 0.734146 | outback |
taurus | 0.884783 | 0.233803 | 0.691639 | 0.725398 | taurus |
mustang | 0.693038 | 0.716824 | 0.766937 | 0.490821 | mustang |
index | reliability | cost | competition | halflife | car_names | |
---|---|---|---|---|---|---|
0 | Car1 | 0.776415 | 0.435083 | 0.236151 | 0.169087 | altima |
1 | Car2 | 0.790403 | 0.987459 | 0.370570 | 0.734146 | outback |
2 | Car3 | 0.884783 | 0.233803 | 0.691639 | 0.725398 | taurus |
3 | Car4 | 0.693038 | 0.716824 | 0.766937 | 0.490821 | mustang |
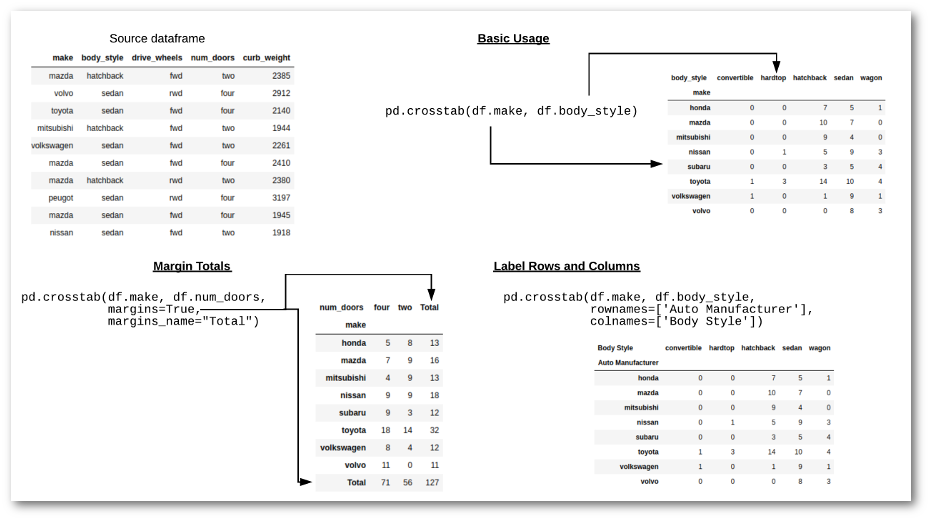
Pandas is an open-source Python library that is powerful and flexible for data analysis. If there is something you want to do with data, the chances are it will be possible in pandas. There are a vast number of possibilities within pandas, but most users find themselves using the same methods time after time. In this article, we compiled the best cheat sheets from across the web, which show you these core methods at a glance.

The primary data structure in pandas is the DataFrame used to store two-dimensional data, along with a label for each corresponding column and row. If you are familiar with Excel spreadsheets or SQL databases, you can think of the DataFrame as being the pandas equivalent. If we take a single column from a DataFrame, we have one-dimensional data. In pandas, this is called a Series. DataFrames can be created from scratch in your code, or loaded into Python from some external location, such as a CSV. This is often the first stage in any data analysis task. We can then do any number of things with our DataFrame in Pandas, including removing or editing values, filtering our data, or combining this DataFrame with another DataFrame. Each line of code in these cheat sheets lets you do something different with a DataFrame. Also, if you are coming from an Excel background, you will enjoy the performance pandas has to offer. After you get over the learning curve, you will be even more impressed with the functionality.
Whether you are already familiar with pandas and are looking for a handy reference you can print out, or you have never used pandas and are looking for a resource to help you get a feel for the library- there is a cheat sheet here for you!
1. The Most Comprehensive Cheat Sheet
This one is from the pandas guys, so it makes sense that this is a comprehensive and inclusive cheat sheet. It covers the vast majority of what most pandas users will ever need to do to a DataFrame. Have you already used pandas for a little while? And are you looking to up your game? This is your cheat sheet! However, if you are newer to pandas and this cheat sheet is a bit overwhelming, don’t worry! You definitely don’t need to understand everything in this cheat sheet to get started. Instead, check out the next cheat sheet on this list.
2. The Beginner’s Cheat Sheet
Dataquest is an online platform that teaches Data Science using interactive coding challenges. I love this cheat sheet they have put together. It has everything the pandas beginner needs to start using pandas right away in a friendly, neat list format. It covers the bare essentials of each stage in the data analysis process:
- Importing and exporting your data from an Excel file, CSV, HTML table or SQL database
- Cleaning your data of any empty rows, changing data formats to allow for further analysis or renaming columns
- Filtering your data or removing anomalous values
- Different ways to view the data and see it’s dimensions
- Selecting any combination of columns and rows within the DataFrame using loc and iloc
- Using the .apply method to apply a formula to a particular column in the DataFrame
- Creating summary statistics for columns in the DataFrame. This includes the median, mean and standard deviation
- Combining DataFrames
3. The Excel User’s Cheat Sheet
Ok, this isn’t quite a cheat sheet, it’s more of an entire manifesto on the pandas DataFrame! If you have a little time on your hands, this will help you get your head around some of the theory behind DataFrames. It will take you all the way from loading in your trusty CSV from Microsoft Excel to viewing your data in Jupyter and handling the basics. The article finishes off by using the DataFrame to create a histogram and bar chart. For migrating your spreadsheet work from Excel to pandas, this is a fantastic guide. It will teach you how to perform many of the Excel basics in pandas. If you are also looking for how to perform the pandas equivalent of a VLOOKUP in Excel, check out Shane’s article on the merge method.
4. The Most Beautiful Cheat Sheet
If you’re more of a visual learner, try this cheat sheet! Many common pandas tasks have intricate, color-coded illustrations showing how the operation works. On page 3, there is a fantastic section called ‘Computation with Series and DataFrames’, which provides an intuitive explanation for how DataFrames work and shows how the index is used to align data when DataFrames are combined and how element-wise operations work in contrast to operations which work on each row or column. At 8 pages long, it’s more of a booklet than a cheat sheet, but it can still make for a great resource!
5. The Best Machine Learning Cheat Sheet
Much like the other cheat sheets, there is comprehensive coverage of the pandas basic in here. So, that includes filtering, sorting, importing, exploring, and combining DataFrames. However, where this Cheat Sheet differs is that it finishes off with an excellent section on scikit-learn, Python’s machine learning library. In this section, the DataFrame is used to train a machine learning model. This cheat sheet will be perfect for anybody who is already familiar with machine learning and is transitioning from a different technology, such as R.
6. The Most Compact Cheat Sheet
Data Camp is an online platform that teaches Data Science with videos and coding exercises. They have made cheat sheets on a bunch of the most popular Python libraries, which you can also check out here. This cheat sheet nicely introduces the DataFrame, and then gives a quick overview of the basics. Unfortunately, it doesn’t provide any information on the various ways you can combine DataFrames, but it does all fit on one page and looks great. So, if you are looking to stick a pandas cheat sheet on your bedroom wall and nail home the basics, this one might be for you! The cheat sheet finishes with a small section introducing NaN values, which come from NumPy. These indicate a null value and arise when the indices of two Series don’t quite match up in this case.
7. The Best Statistics Cheat Sheet
While there aren’t any pictures to be found in this sheet, it is an incredibly detailed set of notes on the pandas DataFrame. This cheat shines with its complete section on time series and statistics. There are methods for calculating covariance, correlation, and regression here. So, if you are using pandas for some advanced statistics or any kind of scientific work, this is going to be your cheat sheet.
Where to go from here?
For just automating a few tedious tasks at work, or using pandas to replace your crashing Excel spreadsheet, everything covered in these cheat sheets should be entirely sufficient for your purposes.
Pandas Cheat Sheet For Data Analysis
If you are looking to use pandas for Data Science, then you are only going to be limited by your knowledge of statistics and probability. This is the area that most people lack when they try to enter this field. I highly recommend checking out Think Stats by Allen B Downey, which provides an introduction to statistics using Python.
Pandas Cheat Sheet Data Science
For those a little more advanced, looking to do some machine learning, you will want to start taking a look at the scikit-learn library. Data Camp has a great cheat sheet for this. You will also want to pick up a linear algebra textbook to understand the theory of machine learning. For something more practical, perhaps give the famous Kaggle Titanic machine learning competition.
Learning about pandas has many uses, and can be interesting simply for its own sake. However, Python is massively in demand right now, and for that reason, it is a high-income skill. At any given time, there are thousands of people searching for somebody to solve their problems with Python. So, if you are looking to use Python to work as a freelancer, then check out the Finxter Python Freelancer Course. This provides the step by step path to go from nothing to earning a full-time income with Python in a few months, and gives you the tools to become a six-figure developer!
Python For Data Science Cheat Sheet
Related Posts
